E Commerce Sales Forecasting
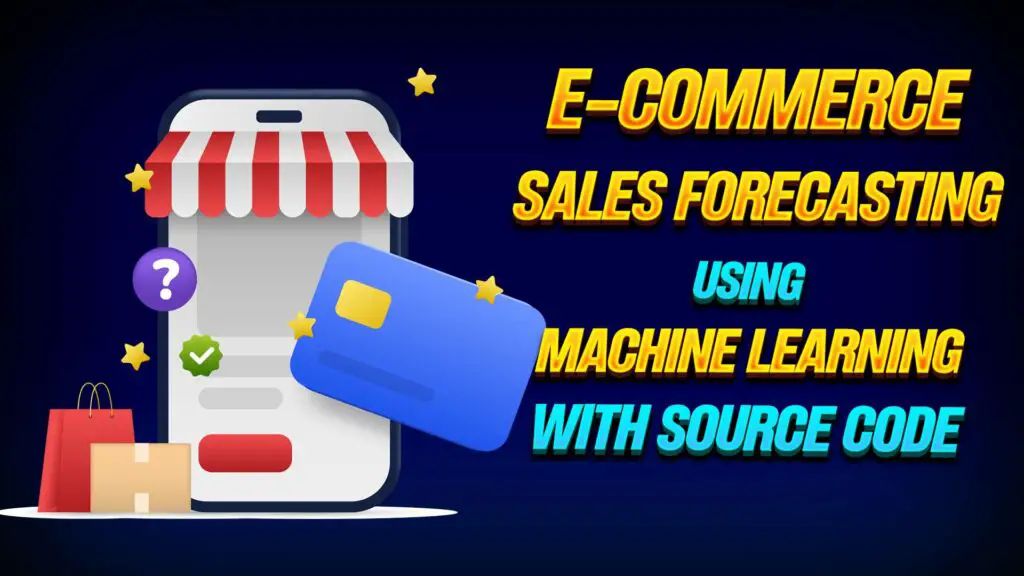
The ecommerce market is growing, but it is essential to be ahead of competitors to succeed. Machine learning is one of the most innovative technologies that has changed the business world. This article explains how an ecommerce sales forecasting system can help businesses and give them a competitive edge. You will also learn how to develop an ecommerce sales forecasting system using a machine learning algorithm. I will also provide source code to help you build e-commerce sales predictions. However, you should know how this system works when developing an e-commerce sales forecasting system.
Understanding the Basics of E-commerce Sales Forecasting
An E-commerce sales forecasting system predicts future sales based on market trends, historical data, and many other factors. By traditional methods, it is very complex to predict sales because the ecommerce market is seasonal and tends to keep changing. Here comes machine learning, which will transform the business and help it grow.
The Evolution of Machine Learning in E-commerce
Machine learning and AI rapidly evolve, and machine learning algorithms have grown significantly. Machine learning provides various tools for ecommerce businesses to analyze vast datasets. ML algorithms have become more advanced, allowing accurate prediction and insights.
Key Components of E-commerce Sales Forecasting Models
E-commerce sales prediction models usually use a mix of past sales data, customer behaviour, seasonality, and outside factors such as economic trends. Machine learning algorithms analyse this data and find patterns that people might miss. This method makes estimates more accurate, which helps businesses better use their resources.
Benefits of Using Machine Learning in E-commerce Sales Forecasting
Improved Accuracy
ML models can examine a lot of data and find small patterns and trends that traditional approaches might miss. This makes it easier to predict sales, so you don’t have to worry about having too much or too little stock. However, extensive data will help you in getting better accuracy in prediction.
Real-time Insights
ML algorithms can handle and analyze data in real-time, allowing businesses to know what’s happening. This skill is essential in the rapidly changing e-commerce environment, where market conditions can change quickly.
Personalized Recommendations
ML models can look at how customers act and what they like, which allows brands to make personalized product suggestions. This makes the experience better for the customer and makes it more likely that they will buy something.
Implementing Machine Learning for E-commerce Sales Forecasting
Data Collection and Preparation
The first step is to gather valuable data. To make reasonable estimates, ML models need clean and well-organized data. It would help if you looked at past sales data, contacts with customers, and external factors.
Choosing the Right Algorithm
ML algorithms are flexible and can work with various data and business needs. To choose the best algorithm, it is better to know the advantages and disadvantages of each algorithm.
Training the Model
To learn patterns and trends, ML models need to be instructed with data from the past. The model can make more accurate predictions when the training data is more accurate.
Continuous Monitoring and Updating
The market changes all the time, and so does e-commerce. Regularly adding new data to the ML model, predictions remain accurate and valuable.
Real-world Examples of ML in E-commerce Sales Forecasting
Amazon’s Recommendation Engine: Amazon uses machine learning to look at how customers behave and what products they like, then makes personalized product suggestions. This not only makes the experience better for users, but it also dramatically increases sales.
Walmart’s Inventory Management: Walmart uses machine learning to handle its inventory, which adjusts stock levels based on past data and insights gained in real-time. Because of this, prices have gone down, and efficiency has gone up.
E Commerce Sales Forecasting using Machine Learning
To make E commerce sales system. First of all download dataset from here.
Data Loading and Libraries:
- Imports necessary libraries for data analysis and machine learning.
- Loads e-commerce data from a CSV file using Pandas.
Data Cleaning and Preprocessing:
- Handles missing values, converts date columns, and deals with canceled invoices.
- Cleans and filters data based on stock codes, descriptions, and customer information.
Exploratory Data Analysis (EDA):
- Explores and visualizes various aspects of the data, such as stock codes, descriptions, customer behaviors, and geographical distribution.
Feature Engineering:
- Derives additional features related to daily product sales, revenue, and temporal patterns.
- Explores and preprocesses unit prices and quantities.
Model Development:
- Utilizes the CatBoost regression model for predicting product sales.
- Defines hyperparameters and performs hyperparameter tuning using Bayesian Optimization.
Time Series Cross-Validation:
- Implements time series cross-validation to evaluate model performance over different time periods.
Multiple Model Management:
- Defines a class for managing multiple CatBoost models.
- Trains and evaluates models using various features and hyperparameters.
Visualization and Interpretability:
- Utilizes SHAP (SHapley Additive exPlanations) for interpretability.
- Visualizes model results, hyperparameter optimization, and feature importance.
E Commerce Sales Forecasting using Machine Learning with source code
## Warehouse optimization
import numpy as np
import pandas as pd
import matplotlib.pyplot as plt
import seaborn as sns
sns.set()
from catboost import CatBoostRegressor, Pool, cv
from catboost import MetricVisualizer
from sklearn.model_selection import TimeSeriesSplit
from sklearn.preprocessing import StandardScaler
from sklearn.cluster import KMeans
from scipy.stats import boxcox
from os import listdir
import warnings
warnings.filterwarnings("ignore", category=DeprecationWarning)
warnings.filterwarnings("ignore", category=UserWarning)
warnings.filterwarnings("ignore", category=RuntimeWarning)
warnings.filterwarnings("ignore", category=FutureWarning)
import shap
shap.initjs()
data = pd.read_csv("../input/ecommerce-data/data.csv", encoding="ISO-8859-1", dtype={'CustomerID': str})
data.shape
data[data.Description.isnull()].head()
data[data.Description.isnull()].CustomerID.isnull().value_counts()
data[data.Description.isnull()].UnitPrice.value_counts()
data[data.CustomerID.isnull()].head()
data.loc[data.CustomerID.isnull(), ["UnitPrice", "Quantity"]].describe()
data.loc[data.Description.isnull()==False, "lowercase_descriptions"] = data.loc[
data.Description.isnull()==False,"Description"
].apply(lambda l: l.lower())
data.lowercase_descriptions.dropna().apply(
lambda l: np.where("nan" in l, True, False)
).value_counts()
data.lowercase_descriptions.dropna().apply(
lambda l: np.where("" == l, True, False)
).value_counts()
data.loc[data.lowercase_descriptions.isnull()==False, "lowercase_descriptions"] = data.loc[
data.lowercase_descriptions.isnull()==False, "lowercase_descriptions"
].apply(lambda l: np.where("nan" in l, None, l))
data = data.loc[(data.CustomerID.isnull()==False) & (data.lowercase_descriptions.isnull()==False)].copy()
data.isnull().sum().sum()
### The Time period <a class="anchor" id="timeperiod"></a>
data["InvoiceDate"] = pd.to_datetime(data.InvoiceDate, cache=True)
data.InvoiceDate.max() - data.InvoiceDate.min()
print("Datafile starts with timepoint {}".format(data.InvoiceDate.min()))
print("Datafile ends with timepoint {}".format(data.InvoiceDate.max()))
### The invoice number <a class="anchor" id="invoiceno"></a>
data.InvoiceNo.nunique()
data["IsCancelled"]=np.where(data.InvoiceNo.apply(lambda l: l[0]=="C"), True, False)
data.IsCancelled.value_counts() / data.shape[0] * 100
data.loc[data.IsCancelled==True].describe()
data = data.loc[data.IsCancelled==False].copy()
data = data.drop("IsCancelled", axis=1)
### Stockcodes <a class="anchor" id="stockcodes"></a>
data.StockCode.nunique()
stockcode_counts = data.StockCode.value_counts().sort_values(ascending=False)
fig, ax = plt.subplots(2,1,figsize=(20,15))
sns.barplot(stockcode_counts.iloc[0:20].index,
stockcode_counts.iloc[0:20].values,
ax = ax[0], palette="Oranges_r")
ax[0].set_ylabel("Counts")
ax[0].set_xlabel("Stockcode")
ax[0].set_title("Which stockcodes are most common?");
sns.distplot(np.round(stockcode_counts/data.shape[0]*100,2),
kde=False,
bins=20,
ax=ax[1], color="Orange")
ax[1].set_title("How seldom are stockcodes?")
ax[1].set_xlabel("% of data with this stockcode")
ax[1].set_ylabel("Frequency");
def count_numeric_chars(l):
return sum(1 for c in l if c.isdigit())
data["StockCodeLength"] = data.StockCode.apply(lambda l: len(l))
data["nNumericStockCode"] = data.StockCode.apply(lambda l: count_numeric_chars(l))
fig, ax = plt.subplots(1,2,figsize=(20,5))
sns.countplot(data["StockCodeLength"], palette="Oranges_r", ax=ax[0])
sns.countplot(data["nNumericStockCode"], palette="Oranges_r", ax=ax[1])
ax[0].set_xlabel("Length of stockcode")
ax[1].set_xlabel("Number of numeric chars in the stockcode");
data.loc[data.nNumericStockCode < 5].lowercase_descriptions.value_counts()
data = data.loc[(data.nNumericStockCode == 5) & (data.StockCodeLength==5)].copy()
data.StockCode.nunique()
data = data.drop(["nNumericStockCode", "StockCodeLength"], axis=1)
data.Description.nunique()
description_counts = data.Description.value_counts().sort_values(ascending=False).iloc[0:30]
plt.figure(figsize=(20,5))
sns.barplot(description_counts.index, description_counts.values, palette="Purples_r")
plt.ylabel("Counts")
plt.title("Which product descriptions are most common?");
plt.xticks(rotation=90);
def count_lower_chars(l):
return sum(1 for c in l if c.islower())
data["DescriptionLength"] = data.Description.apply(lambda l: len(l))
data["LowCharsInDescription"] = data.Description.apply(lambda l: count_lower_chars(l))
fig, ax = plt.subplots(1,2,figsize=(20,5))
sns.countplot(data.DescriptionLength, ax=ax[0], color="Purple")
sns.countplot(data.LowCharsInDescription, ax=ax[1], color="Purple")
ax[1].set_yscale("log")
lowchar_counts = data.loc[data.LowCharsInDescription > 0].Description.value_counts()
plt.figure(figsize=(15,3))
sns.barplot(lowchar_counts.index, lowchar_counts.values, palette="Purples_r")
plt.xticks(rotation=90);
def count_upper_chars(l):
return sum(1 for c in l if c.isupper())
data["UpCharsInDescription"] = data.Description.apply(lambda l: count_upper_chars(l))
data.UpCharsInDescription.describe()
data.loc[data.UpCharsInDescription <=5].Description.value_counts()
data = data.loc[data.UpCharsInDescription > 5].copy()
dlength_counts = data.loc[data.DescriptionLength < 14].Description.value_counts()
plt.figure(figsize=(20,5))
sns.barplot(dlength_counts.index, dlength_counts.values, palette="Purples_r")
plt.xticks(rotation=90);
data.StockCode.nunique()
data.Description.nunique()
data.groupby("StockCode").Description.nunique().sort_values(ascending=False).iloc[0:10]
data.loc[data.StockCode == "23244"].Description.value_counts()
data.CustomerID.nunique()
customer_counts = data.CustomerID.value_counts().sort_values(ascending=False).iloc[0:20]
plt.figure(figsize=(20,5))
sns.barplot(customer_counts.index, customer_counts.values, order=customer_counts.index)
plt.ylabel("Counts")
plt.xlabel("CustomerID")
plt.title("Which customers are most common?");
#plt.xticks(rotation=90);
### Countries <a class="anchor" id="countries"></a>
data.Country.nunique()
country_counts = data.Country.value_counts().sort_values(ascending=False).iloc[0:20]
plt.figure(figsize=(20,5))
sns.barplot(country_counts.index, country_counts.values, palette="Greens_r")
plt.ylabel("Counts")
plt.title("Which countries made the most transactions?");
plt.xticks(rotation=90);
plt.yscale("log")
data.loc[data.Country=="United Kingdom"].shape[0] / data.shape[0] * 100
data["UK"] = np.where(data.Country == "United Kingdom", 1, 0)
### Unit Price <a class="anchor" id="unitprice"></a>
data.UnitPrice.describe()
data.loc[data.UnitPrice == 0].sort_values(by="Quantity", ascending=False).head()
data = data.loc[data.UnitPrice > 0].copy()
fig, ax = plt.subplots(1,2,figsize=(20,5))
sns.distplot(data.UnitPrice, ax=ax[0], kde=False, color="red")
sns.distplot(np.log(data.UnitPrice), ax=ax[1], bins=20, color="tomato", kde=False)
ax[1].set_xlabel("Log-Unit-Price");
np.exp(-2)
np.exp(3)
np.quantile(data.UnitPrice, 0.95)
data = data.loc[(data.UnitPrice > 0.1) & (data.UnitPrice < 20)].copy()
### Quantities <a class="anchor" id="quantities"></a>
data.Quantity.describe()
fig, ax = plt.subplots(1,2,figsize=(20,5))
sns.distplot(data.Quantity, ax=ax[0], kde=False, color="limegreen");
sns.distplot(np.log(data.Quantity), ax=ax[1], bins=20, kde=False, color="limegreen");
ax[0].set_title("Quantity distribution")
ax[0].set_yscale("log")
ax[1].set_title("Log-Quantity distribution")
ax[1].set_xlabel("Natural-Log Quantity");
np.exp(4)
np.quantile(data.Quantity, 0.95)
data = data.loc[data.Quantity < 55].copy()
data["Revenue"] = data.Quantity * data.UnitPrice
data["Year"] = data.InvoiceDate.dt.year
data["Quarter"] = data.InvoiceDate.dt.quarter
data["Month"] = data.InvoiceDate.dt.month
data["Week"] = data.InvoiceDate.dt.week
data["Weekday"] = data.InvoiceDate.dt.weekday
data["Day"] = data.InvoiceDate.dt.day
data["Dayofyear"] = data.InvoiceDate.dt.dayofyear
data["Date"] = pd.to_datetime(data[['Year', 'Month', 'Day']])
grouped_features = ["Date", "Year", "Quarter","Month", "Week", "Weekday", "Dayofyear", "Day",
"StockCode"]
daily_data = pd.DataFrame(data.groupby(grouped_features).Quantity.sum(),
columns=["Quantity"])
daily_data["Revenue"] = data.groupby(grouped_features).Revenue.sum()
daily_data = daily_data.reset_index()
daily_data.head(5)
daily_data.loc[:, ["Quantity", "Revenue"]].describe()
low_quantity = daily_data.Quantity.quantile(0.01)
high_quantity = daily_data.Quantity.quantile(0.99)
print((low_quantity, high_quantity))
low_revenue = daily_data.Revenue.quantile(0.01)
high_revenue = daily_data.Revenue.quantile(0.99)
print((low_revenue, high_revenue))
samples = daily_data.shape[0]
daily_data = daily_data.loc[
(daily_data.Quantity >= low_quantity) & (daily_data.Quantity <= high_quantity)]
daily_data = daily_data.loc[
(daily_data.Revenue >= low_revenue) & (daily_data.Revenue <= high_revenue)]
samples - daily_data.shape[0]
fig, ax = plt.subplots(1,2,figsize=(20,5))
sns.distplot(daily_data.Quantity.values, kde=True, ax=ax[0], color="Orange", bins=30);
sns.distplot(np.log(daily_data.Quantity.values), kde=True, ax=ax[1], color="Orange", bins=30);
ax[0].set_xlabel("Number of daily product sales");
ax[0].set_ylabel("Frequency");
ax[0].set_title("How many products are sold per day?");
## How to predict daily product sales? <a class="anchor" id="model"></a>
# $$ E = \sqrt{ \frac{1}{N}\sum_{n=1}^{N} (t_{n} - y_{n})^{2}}$$
class CatHyperparameter:
def __init__(self,
loss="RMSE",
metric="RMSE",
iterations=1000,
max_depth=4,
l2_leaf_reg=3,
#learning_rate=0.5,
seed=0):
self.loss = loss,
self.metric = metric,
self.max_depth = max_depth,
self.l2_leaf_reg = l2_leaf_reg,
#self.learning_rate = learning_rate,
self.iterations=iterations
self.seed = seed
class Catmodel:
def __init__(self, name, params):
self.name = name
self.params = params
def set_data_pool(self, train_pool, val_pool):
self.train_pool = train_pool
self.val_pool = val_pool
def set_data(self, X, y, week):
cat_features_idx = np.where(X.dtypes != np.float)[0]
x_train, self.x_val = X.loc[X.Week < week], X.loc[X.Week >= week]
y_train, self.y_val = y.loc[X.Week < week], y.loc[X.Week >= week]
self.train_pool = Pool(x_train, y_train, cat_features=cat_features_idx)
self.val_pool = Pool(self.x_val, self.y_val, cat_features=cat_features_idx)
def prepare_model(self):
self.model = CatBoostRegressor(
loss_function = self.params.loss[0],
random_seed = self.params.seed,
logging_level = 'Silent',
iterations = self.params.iterations,
max_depth = self.params.max_depth[0],
#learning_rate = self.params.learning_rate[0],
l2_leaf_reg = self.params.l2_leaf_reg[0],
od_type='Iter',
od_wait=40,
train_dir=self.name,
has_time=True
)
def learn(self, plot=False):
self.prepare_model()
self.model.fit(self.train_pool, eval_set=self.val_pool, plot=plot);
print("{}, early-stopped model tree count {}".format(
self.name, self.model.tree_count_
))
def score(self):
return self.model.score(self.val_pool)
def show_importances(self, kind="bar"):
explainer = shap.TreeExplainer(self.model)
shap_values = explainer.shap_values(self.val_pool)
if kind=="bar":
return shap.summary_plot(shap_values, self.x_val, plot_type="bar")
return shap.summary_plot(shap_values, self.x_val)
def get_val_results(self):
self.results = pd.DataFrame(self.y_val)
self.results["prediction"] = self.predict(self.x_val)
self.results["error"] = np.abs(
self.results[self.results.columns.values[0]].values - self.results.prediction)
self.results["Month"] = self.x_val.Month
self.results["SquaredError"] = self.results.error.apply(lambda l: np.power(l, 2))
def show_val_results(self):
self.get_val_results()
fig, ax = plt.subplots(1,2,figsize=(20,5))
sns.distplot(self.results.error, ax=ax[0])
ax[0].set_xlabel("Single absolute error")
ax[0].set_ylabel("Density")
self.median_absolute_error = np.median(self.results.error)
print("Median absolute error: {}".format(self.median_absolute_error))
ax[0].axvline(self.median_absolute_error, c="black")
ax[1].scatter(self.results.prediction.values,
self.results[self.results.columns[0]].values,
c=self.results.error, cmap="RdYlBu_r", s=1)
ax[1].set_xlabel("Prediction")
ax[1].set_ylabel("Target")
return ax
def get_monthly_RMSE(self):
return self.results.groupby("Month").SquaredError.mean().apply(lambda l: np.sqrt(l))
def predict(self, x):
return self.model.predict(x)
def get_dependence_plot(self, feature1, feature2=None):
explainer = shap.TreeExplainer(self.model)
shap_values = explainer.shap_values(self.val_pool)
if feature2 is None:
return shap.dependence_plot(
feature1,
shap_values,
self.x_val,
)
else:
return shap.dependence_plot(
feature1,
shap_values,
self.x_val,
interaction_index=feature2
)
import GPyOpt
class Hypertuner:
def __init__(self, model, max_iter=10, max_time=10,max_depth=6, max_l2_leaf_reg=20):
self.bounds = [{'name': 'depth','type': 'discrete','domain': (1,max_depth)},
{'name': 'l2_leaf_reg','type': 'discrete','domain': (1,max_l2_leaf_reg)}]
self.model = model
self.max_iter=max_iter
self.max_time=max_time
self.best_depth = None
self.best_l2_leaf_reg = None
def objective(self, params):
params = params[0]
params = CatHyperparameter(
max_depth=params[0],
l2_leaf_reg=params[1]
)
self.model.params = params
self.model.learn()
return self.model.score()
def learn(self):
np.random.seed(777)
optimizer = GPyOpt.methods.BayesianOptimization(
f=self.objective, domain=self.bounds,
acquisition_type ='EI',
acquisition_par = 0.2,
exact_eval=True)
optimizer.run_optimization(self.max_iter, self.max_time)
optimizer.plot_convergence()
best = optimizer.X[np.argmin(optimizer.Y)]
self.best_depth = best[0]
self.best_l2_leaf_reg = best[1]
print("Optimal depth is {} and optimal l2-leaf-reg is {}".format(self.best_depth, self.best_l2_leaf_reg))
print('Optimal RMSE:', np.min(optimizer.Y))
def retrain_catmodel(self):
params = CatHyperparameter(
max_depth=self.best_depth,
l2_leaf_reg=self.best_l2_leaf_reg
)
self.model.params = params
self.model.learn(plot=True)
return self.model
class CatFamily:
def __init__(self, params, X, y, n_splits=2):
self.family = {}
self.cat_features_idx = np.where(X.dtypes != np.float)[0]
self.X = X.values
self.y = y.values
self.n_splits = n_splits
self.params = params
def set_validation_strategy(self):
self.cv = TimeSeriesSplit(max_train_size = None,
n_splits = self.n_splits)
self.gen = self.cv.split(self.X)
def get_split(self):
train_idx, val_idx = next(self.gen)
x_train, x_val = self.X[train_idx], self.X[val_idx]
y_train, y_val = self.y[train_idx], self.y[val_idx]
train_pool = Pool(x_train, y_train, cat_features=self.cat_features_idx)
val_pool = Pool(x_val, y_val, cat_features=self.cat_features_idx)
return train_pool, val_pool
def learn(self):
self.set_validation_strategy()
self.model_names = []
self.model_scores = []
for split in range(self.n_splits):
name = 'Model_cv_' + str(split) + '/'
train_pool, val_pool = self.get_split()
self.model_names.append(name)
self.family[name], score = self.fit_catmodel(name, train_pool, val_pool)
self.model_scores.append(score)
def fit_catmodel(self, name, train_pool, val_pool):
cat = Catmodel(name, train_pool, val_pool, self.params)
cat.prepare_model()
cat.learn()
score = cat.score()
return cat, score
def score(self):
return np.mean(self.model_scores)
def show_learning(self):
widget = MetricVisualizer(self.model_names)
widget.start()
def show_importances(self):
name = self.model_names[-1]
cat = self.family[name]
explainer = shap.TreeExplainer(cat.model)
shap_values = explainer.shap_values(cat.val_pool)
return shap.summary_plot(shap_values, X, plot_type="bar")
daily_data.head()
week = daily_data.Week.max() - 2
print("Validation after week {}".format(week))
print("Validation starts at timepoint {}".format(
daily_data[daily_data.Week==week].Date.min()
))
X = daily_data.drop(["Quantity", "Revenue", "Date"], axis=1)
daily_data.Quantity = np.log(daily_data.Quantity)
y = daily_data.Quantity
params = CatHyperparameter()
model = Catmodel("baseline", params)
model.set_data(X,y, week)
model.learn(plot=True)
model.score()
model.show_val_results();
model.show_importances()
model.show_importances(kind=None)
np.mean(np.abs(np.exp(model.results.prediction) - np.exp(model.results.Quantity)))
np.median(np.abs(np.exp(model.results.prediction) - np.exp(model.results.Quantity)))
products = pd.DataFrame(index=data.loc[data.Week < week].StockCode.unique(), columns = ["MedianPrice"])
products["MedianPrice"] = data.loc[data.Week < week].groupby("StockCode").UnitPrice.median()
products["MedianQuantities"] = data.loc[data.Week < week].groupby("StockCode").Quantity.median()
products["Customers"] = data.loc[data.Week < week].groupby("StockCode").CustomerID.nunique()
products["DescriptionLength"] = data.loc[data.Week < week].groupby("StockCode").DescriptionLength.median()
#products["StockCode"] = products.index.values
org_cols = np.copy(products.columns.values)
products.head()
for col in org_cols:
if col != "StockCode":
products[col] = boxcox(products[col])[0]
fig, ax = plt.subplots(1,3,figsize=(20,5))
ax[0].scatter(products.MedianPrice.values, products.MedianQuantities.values,
c=products.Customers.values, cmap="coolwarm_r")
ax[0].set_xlabel("Boxcox-Median-UnitPrice")
ax[0].set_ylabel("Boxcox-Median-Quantities")
X = products.values
scaler = StandardScaler()
X = scaler.fit_transform(X)
km = KMeans(n_clusters=30)
products["cluster"] = km.fit_predict(X)
daily_data["ProductType"] = daily_data.StockCode.map(products.cluster)
daily_data.ProductType = daily_data.ProductType.astype("object")
daily_data.head()
## Baseline for product types
daily_data["KnownStockCodeUnitPriceMedian"] = daily_data.StockCode.map(
data.groupby("StockCode").UnitPrice.median())
known_price_iqr = data.groupby("StockCode").UnitPrice.quantile(0.75)
known_price_iqr -= data.groupby("StockCode").UnitPrice.quantile(0.25)
daily_data["KnownStockCodeUnitPriceIQR"] = daily_data.StockCode.map(known_price_iqr)
to_group = ["StockCode", "Year", "Month", "Week", "Weekday"]
daily_data = daily_data.set_index(to_group)
daily_data["KnownStockCodePrice_WW_median"] = daily_data.index.map(
data.groupby(to_group).UnitPrice.median())
daily_data["KnownStockCodePrice_WW_mean"] = daily_data.index.map(
data.groupby(to_group).UnitPrice.mean().apply(lambda l: np.round(l, 2)))
daily_data["KnownStockCodePrice_WW_std"] = daily_data.index.map(
data.groupby(to_group).UnitPrice.std().apply(lambda l: np.round(l, 2)))
daily_data = daily_data.reset_index()
daily_data.head()
plt.figure(figsize=(20,5))
plt.plot(daily_data.groupby("Date").Quantity.sum(), marker='+', c="darkorange")
plt.plot(daily_data.groupby("Date").Quantity.sum().rolling(window=30, center=True).mean(),
c="red")
plt.xticks(rotation=90);
plt.title("How many quantities are sold per day over the given time?");
fig, ax = plt.subplots(1,2,figsize=(20,5))
weekdays = ["Monday", "Tuesday", "Wednesday", "Thursday", "Friday", "Saturday", "Sunday"]
yearmonth = ["Dec-2010", "Jan-2011", "Feb-2011", "Mar-2011", "Apr-2011", "May-2011",
"Jun-2011", "Jul-1011", "Aug-2011", "Sep-2011", "Oct-2011", "Nov-2011",
"Dec-2011"]
daily_data.groupby("Weekday").Quantity.sum().plot(
ax=ax[0], marker='o', label="Quantity", c="darkorange");
ax[0].legend();
ax[0].set_xticks(np.arange(0,7))
ax[0].set_xticklabels(weekdays);
ax[0].set_xlabel("")
ax[0].set_title("Total sales per weekday");
ax[1].plot(daily_data.groupby(["Year", "Month"]).Quantity.sum().values,
marker='o', label="Quantities", c="darkorange");
ax[1].set_xticklabels(yearmonth, rotation=90)
ax[1].set_xticks(np.arange(0, len(yearmonth)))
ax[1].legend();
ax[1].set_title("Total sales per month");
daily_data["PreChristmas"] = (daily_data.Dayofyear <= 358) & (daily_data.Dayofyear >= 243)
for col in ["Weekday", "Month", "Quarter"]:
daily_data = daily_data.set_index(col)
daily_data[col+"Quantity_mean"] = daily_data.loc[daily_data.Week < week].groupby(col).Quantity.mean()
daily_data[col+"Quantity_median"] = daily_data.loc[daily_data.Week < week].groupby(col).Quantity.median()
daily_data[col+"Quantity_mean_median_diff"] = daily_data[col+"Quantity_mean"] - daily_data[col+"Quantity_median"]
daily_data[col+"Quantity_IQR"] = daily_data.loc[
daily_data.Week < week].groupby(col).Quantity.quantile(0.75) - daily_data.loc[
daily_data.Week < week].groupby(col).Quantity.quantile(0.25)
daily_data = daily_data.reset_index()
daily_data.head()
to_group = ["StockCode", "PreChristmas"]
daily_data = daily_data.set_index(to_group)
daily_data["PreChristmasMeanQuantity"] = daily_data.loc[
daily_data.Week < week].groupby(to_group).Quantity.mean().apply(lambda l: np.round(l, 1))
daily_data["PreChristmasMedianQuantity"] = daily_data.loc[
daily_data.Week < week].groupby(to_group).Quantity.median().apply(lambda l: np.round(l, 1))
daily_data["PreChristmasStdQuantity"] = daily_data.loc[
daily_data.Week < week].groupby(to_group).Quantity.std().apply(lambda l: np.round(l, 1))
daily_data = daily_data.reset_index()
for delta in range(1,4):
to_group = ["Week","Weekday","ProductType"]
daily_data = daily_data.set_index(to_group)
daily_data["QuantityProducttypeWeekWeekdayLag_" + str(delta) + "_median"] = daily_data.groupby(
to_group).Quantity.median().apply(lambda l: np.round(l,1)).shift(delta)
daily_data = daily_data.reset_index()
daily_data.loc[daily_data.Week >= (week+delta),
"QuantityProductTypeWeekWeekdayLag_" + str(delta) + "_median"] = np.nan
data["ProductType"] = data.StockCode.map(products.cluster)
daily_data["TransactionsPerProductType"] = daily_data.ProductType.map(data.loc[data.Week < week].groupby("ProductType").InvoiceNo.nunique())
### About countries and customers
delta = 1
to_group = ["Week", "Weekday", "ProductType"]
daily_data = daily_data.set_index(to_group)
daily_data["DummyWeekWeekdayAttraction"] = data.groupby(to_group).CustomerID.nunique()
daily_data["DummyWeekWeekdayMeanUnitPrice"] = data.groupby(to_group).UnitPrice.mean().apply(lambda l: np.round(l, 2))
daily_data["WeekWeekdayAttraction_Lag1"] = daily_data["DummyWeekWeekdayAttraction"].shift(1)
daily_data["WeekWeekdayMeanUnitPrice_Lag1"] = daily_data["DummyWeekWeekdayMeanUnitPrice"].shift(1)
daily_data = daily_data.reset_index()
daily_data.loc[daily_data.Week >= (week + delta), "WeekWeekdayAttraction_Lag1"] = np.nan
daily_data.loc[daily_data.Week >= (week + delta), "WeekWeekdayMeanUnitPrice_Lag1"] = np.nan
daily_data = daily_data.drop(["DummyWeekWeekdayAttraction", "DummyWeekWeekdayMeanUnitPrice"], axis=1)
daily_data["TransactionsPerStockCode"] = daily_data.StockCode.map(
data.loc[data.Week < week].groupby("StockCode").InvoiceNo.nunique())
daily_data.head()
daily_data["CustomersPerWeekday"] = daily_data.Month.map(
data.loc[data.Week < week].groupby("Weekday").CustomerID.nunique())
X = daily_data.drop(["Quantity", "Revenue", "Date", "Year"], axis=1)
y = daily_data.Quantity
params = CatHyperparameter()
model = Catmodel("new_features_1", params)
model.set_data(X,y, week)
model.learn(plot=True)
model.score()
model.show_importances(kind=None)
model.show_val_results();
np.mean(np.abs(np.exp(model.results.prediction) - np.exp(model.results.Quantity)))
np.median(np.abs(np.exp(model.results.prediction) - np.exp(model.results.Quantity)))
search = Hypertuner(model)
Because e-commerce constantly evolves, keeping ahead of the curve can be difficult. However, businesses can navigate the complicated web of ecommerce with the help of machine intelligence. Using machine learning algorithms’ predictive capabilities, e-commerce business owners can discover new methods to develop and enhance their procedures and satisfy customers.
To learn more about E-commerce sales forecasting
Machine learning leverages algorithms that can automatically learn and improve from experience without being explicitly programmed. In contrast, traditional forecasting methods often rely on manual calculations or simplistic statistical models that may struggle to capture the complexity of e-commerce data.
E-commerce sales forecasting with machine learning can incorporate various types of data, including historical sales data, website traffic, customer demographics, product attributes, marketing campaigns, and external factors such as economic indicators and weather patterns.
Some common challenges include data quality issues, the need for specialized talent, model interpretability, computational resource requirements, and organizational resistance to change. Overcoming these challenges requires a strategic approach, involving collaboration across departments and a commitment to continuous improvement.
-
Top 20 Machine Learning Project Ideas for Final Years with Code
-
10 Deep Learning Projects for Final Year in 2024
-
10 Advance Final Year Project Ideas with Source Code
-
Realtime Object Detection
-
AI Music Composer project with source code
-
E Commerce sales forecasting using machine learning
-
Stock market Price Prediction using machine learning
-
30 Final Year Project Ideas for IT Students
-
c++ Projects for beginners
-
Python Projects For Final Year Students With Source Code
-
20 Exiciting Cyber Security Final Year Projects
-
Top 10 Best JAVA Final Year Projects
-
C++ Projects with Source Code
-
Artificial Intelligence Projects For Final Year
-
10 Web Development Projects for beginners
-
How to Download image in HTML
-
Fake news detection using machine learning source code
-
How to Host HTML website for free?
-
Hate Speech Detection Using Machine Learning
-
Credit Card Fraud detection using machine learning
-
15 Exciting Blockchain Project Ideas with Source Code
-
Hand Gesture Recognition in python
-
10 advanced JavaScript project ideas for experts in 2024
-
Best Machine Learning Final Year Project
-
Best 21 Projects Using HTML, CSS, Javascript With Source Code
-
Data Science Projects with Source Code
-
Plant Disease Detection using Machine Learning
-
Ethical Hacking Projects
-
Artificial Intelligence Projects for the Final Year
-
20 Advance IOT Projects For Final Year in 2024
-
Top 7 Cybersecurity Final Year Projects in 2024
-
Python Projects For Beginners with Source Code
-
Phishing website detection using Machine Learning with Source Code
-
17 Easy Blockchain Projects For Beginners
-
portfolio website using javascript
-
Top 13 IOT Projects With Source Code
-
Best 13 IOT Project Ideas For Final Year Students
-
10 Exciting Next.jS Project Ideas
-
Fabric Defect Detection
-
Heart Disease Prediction Using Machine Learning
-
How to Change Color of Text in JavaScript
-
Why Creators Choose YouTube: Exploring the Four Key Reasons
-
10 Exciting C++ projects with source code in 2024
-
Wine Quality Prediction Using Machine Learning
-
Diabetes Prediction Using Machine Learning
-
10 Final Year Projects For Computer Science With Source Code
-
Where Do YouTubers Get Their Music?
-
10 TypeScript Projects With Source Code
-
Chronic Kidney Disease Prediction Using Machine Learning
-
Titanic Survival Prediction Using Machine Learning